A Guide to Data Literacy: How to Interpret Data in Media
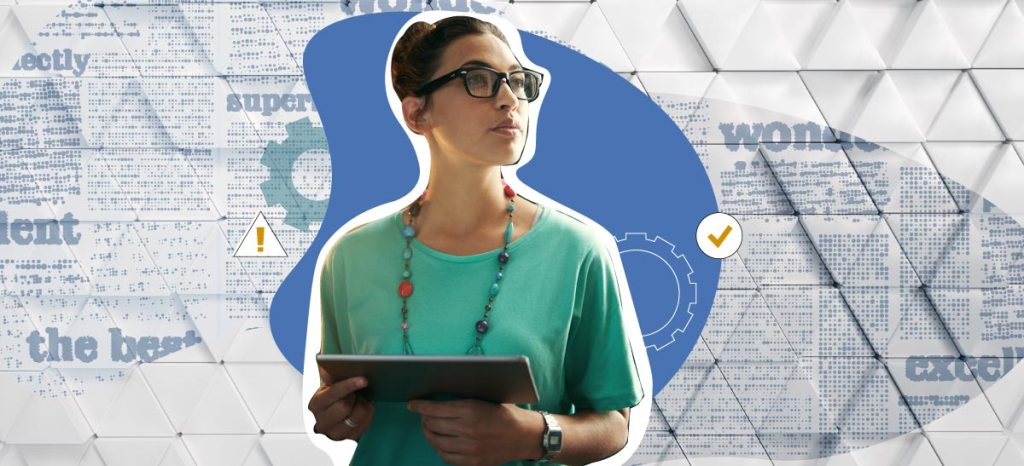
You don’t have to be a full-time data scientist or mathematician to need data literacy. In the digital age, data is all around us, and our consumption of information affects the way we live, work, play, and make decisions about our lives.
“The information has always been around, but for the first time we have a huge data explosion. There are so many different types of data, and so many things are being decided based on that data,” said Amit Bhattacharyya, faculty member at the UC Berkeley School of Information and head of data science at Vox.
A basic level of data literacy is one part of being a well-informed citizen and being able to contextualize information in the news. Understanding the fundamentals of data science can help everyone when browsing the web, reading the news, and applying information to their own lives.
What Is Data Literacy?
Data literacy is the ability to read, write, and communicate data in context and with meaning. This includes developing an understanding of data sources and constructs, analytical methods and techniques applied, and the ability to describe the meaning of the findings.
While the comprehensive definition may seem daunting, data literacy can be broken into three main components:
- Reading data: making sense of quantitative and qualitative information
- Working with data: collecting and organizing data for analysis
- Communicating findings: presenting and applying the information usefully
Realistically, not everyone may need the advanced technical skills of working with data, but Bhattacharyya said data literacy can help people in every professional field make better decisions about their subject matter, whether that be research, work, school, finances, and more.
“You can continue doing the thing that you want to do. You can also incorporate data into it to make it better,” he said.
For example, undergraduate students may benefit from pairing a bachelor’s in data science with psychology, urban planning, or other subjects. “Incorporating a little bit of data science can really help them go down the road of becoming an expert,” he said.
“The problem with the Internet is when you search for something, it doesn’t search and give you reputable results — it just gives you results.”
— Amit Bhattacharyya, faculty member at the UC Berkeley School of Information and head of data science at Vox
Even without a professional application, being data literate can help people harness data to understand the world around them — particularly as data becomes a more prevalent part of news media.
“In the media, there’s a lot of data being thrown at you,” Bhattacharyya said. “The ability to analyze it on the fly is very quick, seemingly magical.”
Whether it’s an article about sports, health care, or politics, “the information’s getting thrown out at you, so what are you supposed to do with it? Data literacy happens in the moment of media literacy, because the two are happening at the same time.”
Following sports statistics, calculating tips, deciding whether to bring an umbrella: these are all ways that people regularly consume data and use it to make decisions for themselves.
The Benefits of Data Literacy
- Making sense of complex or competing information
- Deriving clear conclusions from a set of data
- Estimating realistic solutions for everyday problems
- Applying information to your own life or decisions
- Filtering out unreliable sources with ease
The Risks of Data Illiteracy
- Misusing information to prove an ulterior motive
- Becoming confused about next steps based on a data set
- Struggling to make quick decisions based on estimates
- Spreading unethical or harmful findings to others
- Relying on inaccurate or manipulated information
Important Data Literacy Terms to Know
Even if you’re not a researcher, it’s likely you’ll encounter data concepts that influence your understanding of information. Use the chart below to review terms, definitions, and examples of frequently used terms about data literacy.
Term | Definition | Example |
---|---|---|
Aggregate data | Information that has been compiled into a useful summary. | The names and number of students who graduated from every local high school can be compiled into one school district’s graduation rate. |
Causation | One variable causes another to change, also known as cause and effect. | Too much UV exposure without sunscreen causes skin to become sunburned. |
Correlation | The extent to which two variables are linearly related without directly causing each other to change. | The longer your hair is, the more shampoo you may use, but the amount can also be affected by hair texture, exercise regimen, and hydration. |
Distribution | The arrangement and probability of every possible value for a given variable. | When rolling a die, the possible outcomes are between one and six with an equal likelihood of each. |
Estimation | A rough calculation of the value, amount, or outcome of a specific thing. | Knowing how long it takes to run a mile can help you estimate how long it takes to run a 5K (3.1mi) without using a calculator. |
Margin of error | A degree of error that is calculated when using random samples data collection. The smaller the margin of error, the more accurate and applicable the results are to the general population. | A survey finds 60% of customers are very satisfied with a store’s mask mandate. But the margin of error is 5%, because not every single customer responded to the survey. This means between 55 and 65% of the store’s customers may realistically be very satisfied. |
Population | The people, places, observations, or things that share a characteristic to be studied or researched. | All the people who have earned a bachelor’s degree in the United States in a given year (see also: sample size). |
Primary data | Information collected by researchers through interviews, surveys, or experiments. | A company conducts exit interviews to learn why employees decided to leave the organization (see also: secondary data). |
Probability | The likelihood that a variable will have a given outcome. | People are likely to leave the house without an umbrella when the chance of rain is less than 30%. |
Qualitative data | Information that is not based on numbers but rather descriptive or conceptual variables. | The reason why a patient misses a recurring medical appointment is that public transportation was unreliable in her area (see also: quantitative data). |
Quantitative data | Information based on numerical or otherwise measurable values. | The number of times a patient has missed a medical appointment. |
Results | Data that comes from running an experiment or survey, which can be used to communicate findings. | The results of a survey show that customers are dissatisfied with a store’s lax mask policy, which leads the management to change the policy. |
Sample size | A small group that represents a larger population. | All the people who have graduated from the top 25 universities with a bachelor’s degree in a given year. |
Secondary data | Information aggregated by other sources that are used to make comparisons or inferences. | An occupational therapist compares the exit interviews from several companies to identify reasons why employees change fields or organizations. |
Statistically significant | The probability that a given value is real and not due to random chance alone, which can help account for sampling errors. | The results of a study on a weight loss pill show that the average person lost five pounds, but researchers have to compare the amount to a control group to determine if the difference is due to chance. |
Variables | Elements that can be measured to show correlation, causation, or other relationships among different circumstances. | Researchers studying grocery customers’ purchasing habits must account for demographics such as income, age, and location. |
Strategies for Practicing Good Data Literacy
The pervasive negative stigma against math, which often comes from bad experiences in math courses or a belief that math and science are too difficult, has to be addressed before people feel confident enough to build useful data literacy skills.
People may think they’re bad at math, “but on the other hand, we all implicitly walk around doing a lot of math in our heads every day,” Bhattacharyya said.
The reality is that people are using these principles frequently, which means there’s merit for learning how to use them correctly. For example, most people interact with the news on a daily basis, whether scrolling through articles on social media, reading the newspaper, or chatting with others about big headlines.
[Internet] users still have to be vigilant and consistent about building the data literacy skills to consume and share information responsibly.
Bhattacharyya recommends being intentional and investigative about the data included in news articles.
“The quickest and easiest way is to actually do a little bit of a mini literature scan by yourself right in the moment. Of course, your search engine is filtering, ranking, and organizing the information for you,” he said. “If there’s an obvious consensus among all five of the first articles you see, maybe that’s okay, but at least you want to make sure you’re getting your news from a diversity of places.”
Understanding what makes data authoritative or research reputable comes with understanding the scientific method of research and also looking for specific red flags that inform the outcome of the information.
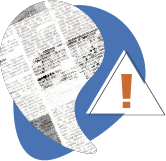
Nine Red Flags for Spotting Misused Data
1
Clickbait Headlines: Does the headline really tell the whole story? What context is missing from the title or the article? Compare multiple articles to each other to identify missing pieces or manipulated information.
2
Source Authority: What organization published the research, and what other types of research do they publish? Check the “About Us” page, and make sure the URL is spelled correctly to avoid fake versions of reputable sites.
3
Reputability: Are other reputable sources reporting about this topic? Are they including similar data points or information about the research? If this is the only site with the information, chances are it’s not real news, or it may be a satirical site.
4
Sponsored Research: What organization or individual is financing the research? What motives might they have for the research to favor their hypothesis? Some news sites also have sponsored content, which means the article is written to favor a particular company and may not go through the same editorial process as regular news.
5
Sample Size: How many people were part of the study or research? Do they accurately represent the larger population being studied? Small samples of a large population are typically not representative or statistically significant findings.
6
Author Attribution: Are the authors or reporters credited with a byline? Is the content labeled as editorial or opinion? Articles without these elements typically need more citation or verification.
7
Manipulated Data: Are the findings being discussed in percentages or percentage points? Have the findings been extrapolated or exaggerated to fit the headline’s needs? Click through to the original source of the study, and see if the data in the findings section matches up with what the outlet wrote.
8
Inflammatory Language: Does the article make you feel angry or suggest that you should? Try reading a few more articles about the topic from other outlets; chances are the inflammatory article includes misleading or manipulative language.
9
Causal Claims: Does the article imply that a study found a direct link between two variables? Is it correlation or causation? Click through to the original research to identify the results and findings from the source.
The more you practice, the easier it will be to use these principles as filters for the way you live every day. People may already have their own sense of which news outlets have angles they agree or disagree with and which ones filter out data from sites that they believe aren’t reputable. But the risk of sticking to a few biased sources may mean they miss out on more reputable information.
“It puts a huge burden on the media consumer to know that this is what Fox News, or The New York Times, or Vox Media is, and have a sense of where they live on the spectrum,” Bhattacharyya said. “Unfortunately, we all have basically this radio tune knob in our head nowadays. You see a piece of news, or a study, or a result, and you have to put it through this new filter that you developed. Say it’s coming from CNN — so now you have to decide: what does that mean to you?”
Practicing data literacy while filtering through the news can also influence the way people share articles on social media. Use the checklist below to help decide whether an article is reputable before sharing:
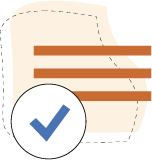
Data Literacy Checklist for Sharing News Articles
Before sharing a news article on your feed or with a friend, read through the full article first, and find the original research cited in the article. Then, use the checklist below to scan for specific elements of reputable data.
- The content of the article matches or supports the headline.
- The source data is linked and easily accessible to readers.
- The source data matches the numbers reported in the article.
- The article cites more than one dataset or study to support the claim.
- The information is being reported by more than one reputable outlet.
If you answered “No” to one or more of these, look for more ways to further verify the information before sharing it.
“The problem with the Internet is when you search for something, it doesn’t search and give you reputable results — it just gives you results,” Bhattacharyya said. Those results are typically based on a person’s search history, demographics, location, and predictive models from the search engine itself.
“People like you like these results better, so we’re going to give you those results,” he explained.
Though the Internet offers so much access to information and has transformed the way people learn about the world, users still have to be vigilant and consistent about building the data literacy skills to consume and share information responsibly.
“We all live in this world now, and maybe we always did. I’m not sure exactly how we get to teach data literacy to everybody, but it certainly seems important enough to try,” Bhattacharyya said.
This concludes Part 1 of “A Guide to Data Literacy.” Visit Part 2, “Strategies for Teaching Students about Data Literacy.”
Citation for this content: datascience@berkeley, the online Master of Information and Data Science from UC Berkeley