How Artificial Intelligence Bias Affects Women and People of Color
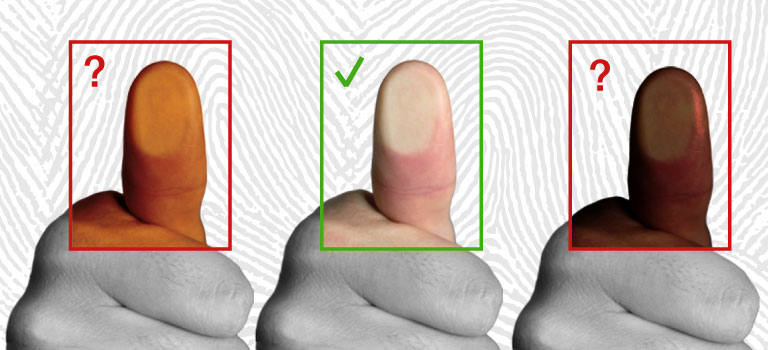
Bias, or prejudice for or against a thing, person, or group, is traditionally thought of as part of human decision-making. But, when left unchecked, bias can extend beyond individual actions and infiltrate the systems created by people designed to protect everyone.
Even computers, which think in purely mathematical terms, take on the human trait of prejudice.
Artificial intelligence (AI) is a computational problem-solving mechanism; data teaches a machine how to make specific decisions and tests performance against an answer key. Eventually, the training wheels are removed and the computer can complete the decision-making process independently on new data. However, the computer can also learn to prefer certain demographics and discriminatory outcomes.
Over the past decade, researchers, lawmakers and engineers have debated not just the technical, but the moral and ethical codes of AI.
The Stanford Encyclopedia of Philosophy outlines some of AI’s ethical issues like opacity, when information about algorithmic processes and results are kept secret, and surveillance, when the data used to train computers is collected without consent or at risk of personal privacy.
These issues contribute to a system of algorithmic bias in which “some algorithms run the risk of replicating and even amplifying human biases, particularly those affecting protected groups.” Research company Gartner predicts that over the next year, 85 percent of AI projects will deliver incorrect outcomes due to some form of algorithmic bias.
Members of marginalized groups, such as women and people of color, are often those adversely affected by erroneous algorithms.
Read on to learn more about how bias enters the algorithmic process, and how it disproportionately affects marginalized groups.
Table of Contents
(Lack of) Representation in AI, at a Glance
- In 2017, just 12 percent of contributors in leading machine learning conferences were women. (WIRED)
- Female AI professionals earn 66 percent of the salaries of their male counterparts. (AI Now Institute)
- Among new AI Ph.D.s in 2019 who are U.S. residents, almost half were white, 22 percent were Asian, 3.2 percent were Hispanic, and 2.4 percent were Black. (Stanford)
- The standard training dataset for facial recognition is reportedly 84 percent white faces and 70 percent male faces. (Jolt Digest)
How Do Algorithms Become Biased?
AI is created using a feedback loop. Real-world experiences shape data, which is used to build algorithms. Those algorithms drive decisions affecting real-world experiences. This kind of circular reasoning means that bias can infiltrate the AI process in many ways.
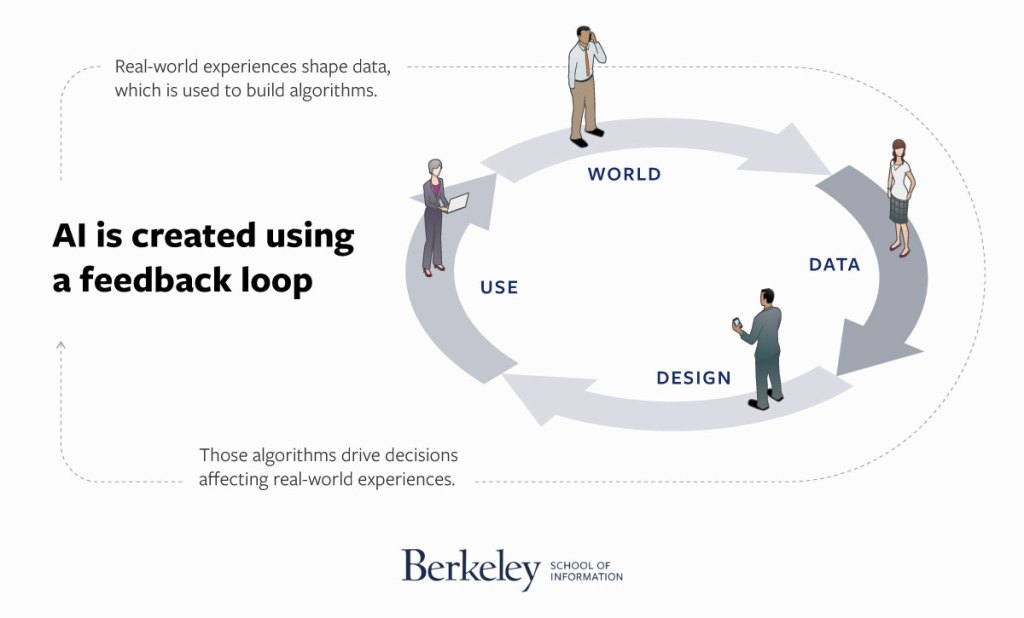
Research in the British Medical Journal on how AI could augment healthcare inequality provides a framework for how bias and discrimination can corrupt the algorithmic process at all stages:
World
Inequality affects outcomes:
Things like institutional racism, discriminatory laws and inequitable access to resources lead to poorer health outcomes for members of minority groups and women.
Data
Discriminatory data:
Collected data can lack fair representation and include sampling biases. Also, the real-world patterns of inequality affect data distribution.
Design
Human bias in AI development:
Developer bias infiltrates algorithmic design, testing, and deployment; demographic imbalances in the field contribute to unfair process selection and methodology.
Use
Application injustices:
Algorithms are applied to the real world in a way that exacerbates inequality and deepens discriminatory gaps.
[Back to World]
Examine the case of an algorithm meant to predict mortgage defaults. Financial institutions automated the risk evaluation portion of the loan application process. Instead of leaving it to an individual, a computer looked at applicants’ credit histories and determined the likelihood of default.
The outcome, according to 2021 research on the default algorithm, is that the computer contributes to unequal credit market outcomes for historically underserved groups. Why?
World
People of color are given fewer loans and have lower home ownership rates.
Data
People of color have less data in their credit history. The resulting statistical noise creates an information disparity that increases uncertainty.
Design
Algorithm creators do not perform an analytical study on the data to search for biases.
Use
Loans for members of minority groups are evaluated as higher risk and affect the likelihood that a bank will approve them.
[Back to World]
These interacting systems — real-world discrimination, industry demographics, and technical processes — each have the potential to drive or mitigate unfair algorithms.
Examples of How AI Bias Affects Marginalized Groups
Facial recognition
Facial recognition software, used in services like biometric security at airports and on phones, performs better on lighter skin tones, according to the Gender Shades project. The study also found that these algorithms — from Microsoft, IBM and Face++ — performed the worst on darker skin-toned females.
Job recruiting
An Amazon job recruiting tool meant to scan resumes and return the most qualified applicants was abandoned in 2015 when engineers discovered it was not gender-neutral, according to Reuters. The algorithm used data from prior applicants, which was skewed toward men.
Healthcare decisions
An algorithm used in U.S. hospitals was supposed to direct patients to healthcare programs, but a 2019 study found it was less likely to refer Black people to the care they need than equally sick white patients. The software, which used healthcare costs in the preceding year as a primary indicator for the seriousness of a disease, did not recognize Black patients were substantially sicker and received less access to care.
Prison sentencing
Algorithms used in the criminal justice system predict the likelihood that those convicted will commit a future crime, which affects their sentence. These “risk assessments” are unreliable. Research from ProPublica found it was 20 percent accurate in predicting crime. But they are also biased: The algorithm is more likely to falsely label Black defendants as future criminals and white defendants as low risk.
Representation in AI
There is not comprehensive data on the prevalence and experiences of marginalized groups in the artificial intelligence community.
Generally, data shows the growth of girls and women entering computing has slowed in the past few decades. Data from the National Center for Education Statistics shows that approximately one in five computer science degree recipients were women in the 2018-19 academic year, compared to more than one in three during the 1985-86 year.
Women of color are even more scarce in the field: Two percent of computer degree recipients were Black women in the 2018-19 academic year.
Specifically within the artificial intelligence industry, data shows women make up a disproportionate minority of professionals, and earn less on average.
12%
of contributors in leading machine learning conferences were women in 2017, according to WIRED data.
22%
of AI professionals are female, according to the World Economic Forum Global Gender Gap Report.
$0.66
The amount that women in AI earn for every dollar their male counterparts make, according to the AI Now Institute’s Discriminating Systems Report (PDF, 474 KB).
Data from Stanford shows people of color are also underrepresented among the highest levels of artificial intelligence education. Among new AI Ph.D.s in 2019 who are U.S. residents, almost half were white and about 22 percent were Asian (PDF, 14 MB). Black and Hispanic PhDs made up 2.4 percent and 3.2 percent of Ph.D. students, respectively.
The lack of representation affects algorithmic outcomes, too. Research from Columbia University shows that biased predictors were partially attributed to the lack of diversity of the group responsible for creating an algorithm (PDF, 78 KB).
Additionally, the data used in algorithms is skewed white and male. Faces in the Wild is considered the standard training dataset for facial recognition, but Jolt Digest reports it is 84 percent white faces and other analysis finds it is about 70 percent male faces.
5 Women and BIPOC Leaders in AI Ethics
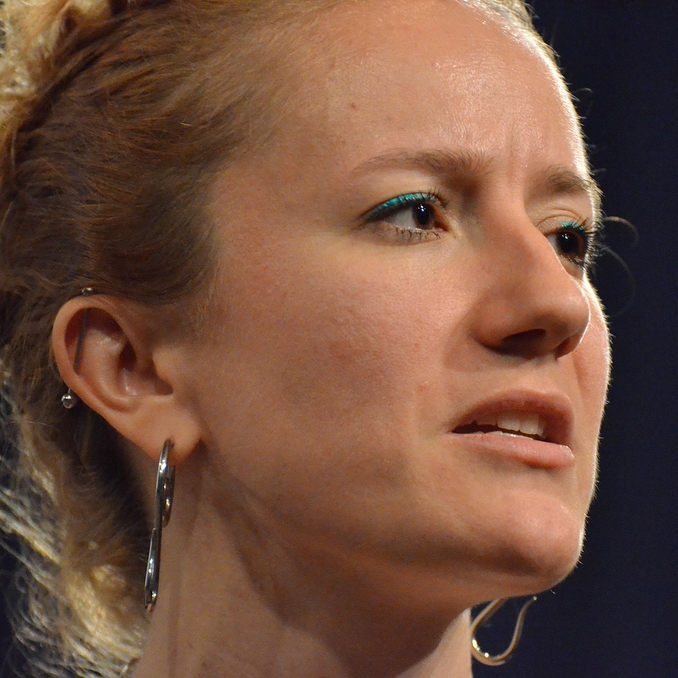
danah boyd
Partner researcher at Microsoft and Data & Society founder
boyd completed her Ph.D. from the UC Berkeley School of Information (I School) in 2008, where her research focused on teenager sociality in networked public spaces like Facebook. In 2014, she founded Data & Society, which studies the societal implications of AI and automation on inequality. In a 2017 video for the Berkman Klein Center, Boyd explains how AI systems can be used to reduce social challenges. Image via danah boyd’s website.
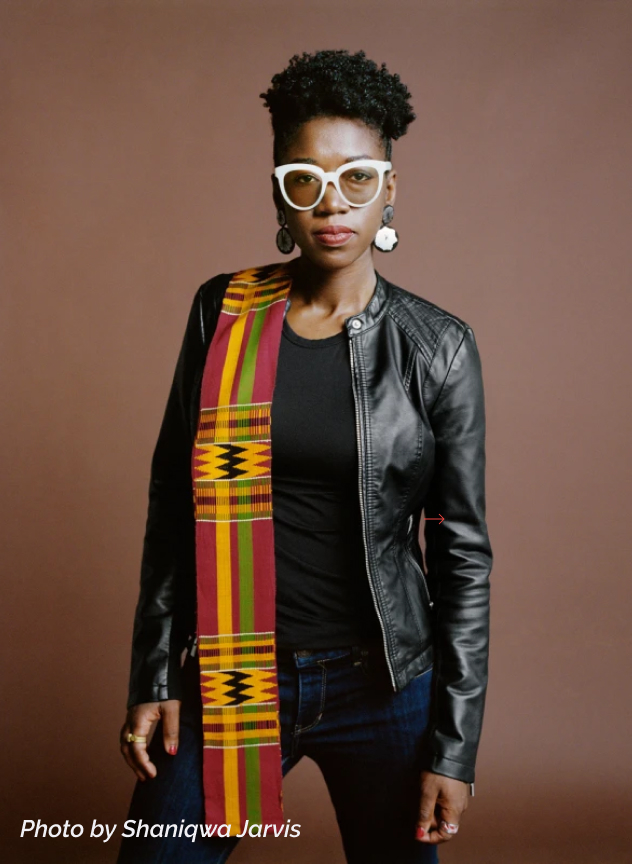
Joy Buolamwini
Algorithmic Justice League founder
Buolamwini’s MIT thesis Gender Shades evaluated gender classification of facial recognition models and uncovered large racial and gender biases in some of the industry’s most prominent services. In 2016, she founded the Algorithmic Justice League (AJL), which focuses on the societal implications and harms of AI. Buolamwini’s Ted Talk “How I’m Fighting Bias in Algorithms” helped launch the AJL. Image via Joy Buolamwini’s website.
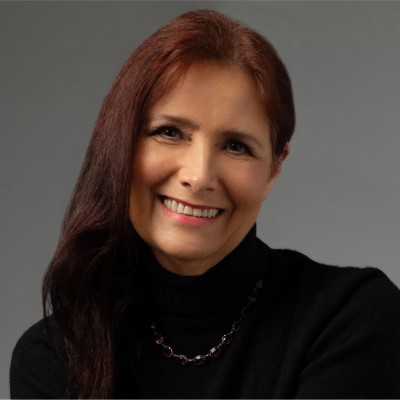
Kay Firth-Butterfield
Head of artificial intelligence and machine learning at the World Economic Forum
Firth-Butterfield was the world’s first chief AI ethics officer and creator of the #AIEthics hashtag on Twitter. She is considered one of the leading experts on the governance of artificial intelligence. Her work at the 2015 Asilomar Conference helped create the first set of ethical guidelines for responsible AI. Image via Kay Firth-Butterfield’s LinkedIn profile.
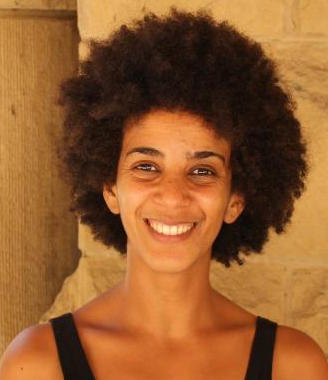
Timnit Gebru
Black in AI founder
Gebru was a co-lead of Google’s ethical artificial intelligence team until December 2020, when the company asked her to retract a paper critical of the company’s technology. Without additional information about the process, Gebru refused. She founded Black in AI in 2017 as a place to foster collaboration and support among Black people in the field of artificial intelligence. Image via Timnit Gebru’s biography.
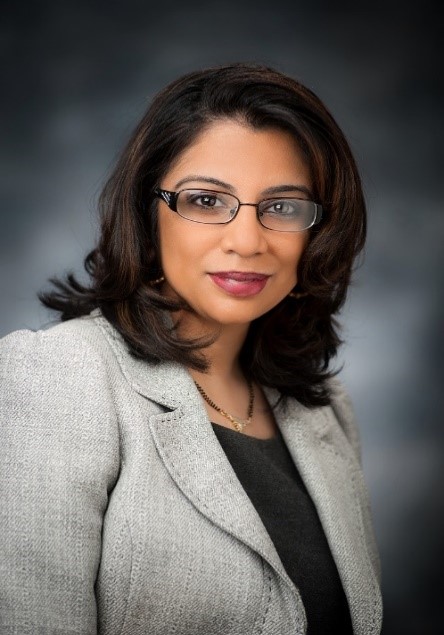
Alka A. Patel
Patel is the inaugural lead of AI Ethics Policy at JAIC, leading the DOD’s efforts on Responsible AI. Before joining DOD, she was the executive director of the Digital Transformation and Innovation Center at Carnegie Mellon University. Image via Alka A. Patel’s ACT-IAC biography.
How to Support Women and People of Color in the AI Community
Tech leaders, managers and colleagues who want to be allies to those in marginalized communities can help foster a more inclusive and welcoming environment. Some of the ways to encourage women and people of color to join, and happily stay, in the tech industry include:
Assess diversity in your own organization to look for opportunities for improvement and learn about employees’ experiences. Forbes recommends evaluating diversity at different levels throughout your company and having conversations with your team about what a culturally competent environment looks like to them.
Invest in employees’ professional development and leadership skills. The Center for Creative Leadership says this will help improve employee retention and build a stronger organization.
Provide employees with access to external communities and time for mentorship opportunities.
Some examples include:
Resources for Women and People of Color in AI
Here are some additional resources for people looking for professional research, projects in progress and learning tools.
AI Ethics Organizations
AI Ethics Additional Resources
- Changing the Curve: Women in Computing, datascience@berkeley
- Artificial Intelligence Strategy Online Short Course, Berkeley I School Online
- Automating Inequality: How High-Tech Tools Profile, Police, and Punish the Poor by Virginia Eubanks
- Weapons of Math Destruction: How Big Data Increases Inequality and Threatens Democracy by Cathy O’Neil
- Atlas of AI by Kate Crawford
- How I’m Fighting Bias in Algorithms, Joy Buolamwini’s Ted Talk
Earn a certificate in Artificial Intelligence Strategy from the UC Berkeley School of Information in just six weeks.