Annie Lane
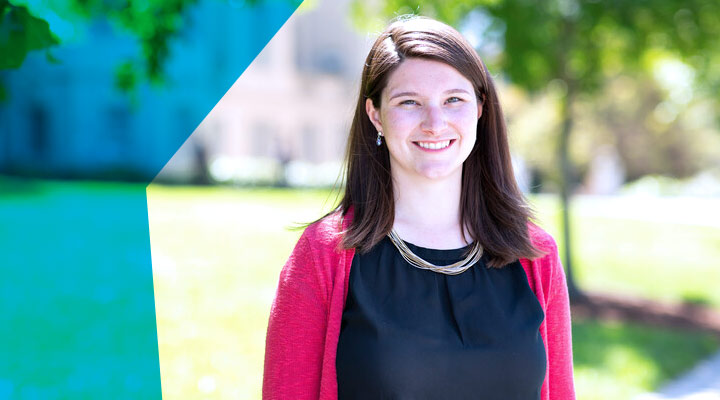
MIDS 2020
Senior Data Scientist
GE Healthcare
How has the program prepared or helped you in your current job?
I currently work in a non-data-native company that has a lot of untapped opportunities to learn from data and improve our customer experience with new data science–inspired features. I’m building the foundations and proving the value of these kinds of efforts while sharing the data science language with my colleagues. I plan to continue spreading data science throughout our organization while applying it to create new efficiencies and capabilities within the health care industry.
What was your favorite course and why?
This is a hard one. I think W241 Experiments and Causality was a fantastic experience. My team conducted an experiment with over 75 volunteers to measure the effect of participation in “Step Competitions” on the amount of steps people take. While it sounded like a straight-forward question to answer, we faced a lot of semantic and logistical questions in the design and execution of the experiment.
A lot of times, we focus on analysis techniques once you have a cleaned-up data set in hand, so it was great to develop an appreciation for the data collection process. Plus, our class had discussions and practiced critical thinking about data quality, which is fundamental to developing models that not just have good “accuracy” or other metrics in a Jupyter Notebook, but depend on robust data to “work” in the real world.
What one piece of advice would you give to prospective or current MIDS students?
When you get curious about something, go explore it. See if you can apply something you’ve learned or seen elsewhere to the domain you’re curious about. There isn’t a prescribed set of steps to get to an impactful output — you’ll probably “fail” along the way, but that’s just learning what will not work so you can focus energy on what may work.